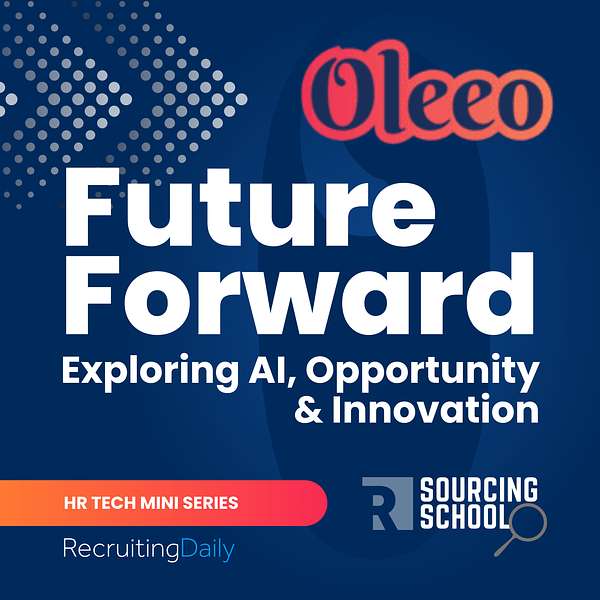
Sourcing School by RecruitingDaily
The team and audience of RecruitingDaily discuss sourcing tools, news, need-to-knows, need-to-use, and a whole lot more. Class is in session.
Sourcing School by RecruitingDaily
Sailing into the Future of HR Tech with George LaRocque of WorkTech
Uncover the transformative power of HR Tech with industry expert George LaRocque, Founder of WorkTech. We dissect AI's impact, from streamlining data integration to uncovering hidden talents in Slack channels. Question what you know about AI, exploring the allure and risks of borrowed language models, comparing the emergence of AI to the rise of social media.
This conversation promises an enlightening exploration of the future of HR Tech, one you don't want to miss.
Special mini series recorded with Oleeo at HR Tech 2023 with hosts Ryan Leary, Brian Fink, and Shally Steckerl
Listen & Subscribe on your favorite platform
Apple | Spotify | Google | Amazon
Visit RecruitingDaily
Twitter @RecruitingDaily
Join the Secret Sourcing Group
Learn more about #HRTX Events
And we are back. This is Shali Stekaral coming at you live from the HR Tech Expo floor Powered today by the Olio booth. We are broadcasting here in the Olio booth, taking names and prisoners and just having a good old time. Our next guest is a long, long time friend, old friend of mine, george LaRocque, and George is from Workforce Tech, work Tech, work Tech, work Tech. How appropriate, hr Tech, work Tech. I think Work Tech is better than HR Tech because, like it's about work.
Speaker 2:Right.
Speaker 1:Yeah, I like it. So, george, why are you here at the show and what's been your impression so far?
Speaker 2:Well, I'm here at the show for a couple of reasons. One, I do a lot of work at the show, so I had a pre-conference bringing together investors and startups and scale-ups that this show puts on, and I'm the chair of that. I've been hosting the startup pitch fest for the last few days.
Speaker 2:Oh you introduce people and everything. Yeah, I do all that. I provide a little insight on, you know, with the data that we track and the trends that we track, all of the investment coming into the space and you know what are the trends, what are people buying, et cetera. You know in between. And then I also come here to meet people, see friends and learn, see what's going on. It's a great place to get a pulse on the tech side of what's happening.
Speaker 1:It is Definitely. So what is your take on the pulse? That's what's happening now. Not the pitch fest, but like looking at the booths and walking around. Yeah.
Speaker 2:So it seems like the two conversations are skills and AI. Of course we knew it.
Speaker 1:There's a bingo word for you. We got ATS, we got AI and we got CRM. Bingo, yeah. So skills meaning like skilling up, learning and development, or like finding skills or ontologies, yeah.
Speaker 2:Well, because everybody's talking about it, yes, all of that and more. You know. So, depending on where they're coming from, you've got even the labor market data. Folks are starting to look at skills market data. So looking at, you know, not just the jobs and geographic location and comp by job, but skill, skills and comp by skill and that sort of thing. And then you've got, as you, you know, start from the very, very, very, very top of the funnel and go all the way into, you know, post hire and learning and development. It's, you know, skills, so marketplaces and upskilling and re-skilling, and it's, you know, everybody's got an angle on skills, got you Everybody.
Speaker 1:So I have two questions for you, all right, based on the skills conversation. The first of those is from a personal perspective. Recently started not recently, it lasts several months I started using LinkedIn's. I don't know skills catalog or ontology so you're probably a lot more familiar with this than I am, but when you are posting jobs to LinkedIn recruiter through the API and, for example, like greenhouse publishes them, you know directly. It used to not be very catalog, so if you had a software engineer, it was just posted as a software engineer. It might have maybe kind of narrowed down by industry. But now they have this big catalog of skills that are interrelated. Are you familiar with what I'm talking about?
Speaker 2:I'm familiar because I've seen the content on it. But I haven't gone deep on that.
Speaker 1:So I started working with that and tweaking kind of what. Because there's two problems that happen very frequently with job postings, and they have a lot to do with classification. One of them is the job title itself. If a company has a let's just call it a cool job title, it might be cool to the company, it might set them apart, it might be unique, but people don't know what it means. And so you end up with candidates that don't understand what the job is and they apply anyway because they're figured out it might as well take a chance and they're not qualified because they're just not really getting the job title. And then the opposite of that happens you got people who are in the job that you want to hire for, but their job title is completely different than your cool title and so they don't see themselves in it. So they might think well, I'm a software engineer and this job is for a you know, back-end data developer or whatever, and that's not me, so they don't apply. So the good ones don't apply because the job title doesn't resonate and the ones that don't match apply because they don't quite understand it. So it's a problem in that you end up with just a higher percentage of not really qualified candidates.
Speaker 1:This has helped combat that, in that these skills are matched, and so I think the confidence level in applicants is increasing Over time. It'll get better. Where a software engineer looks at the job and goes, ah, go-lang, back-end data scientist developer, that doesn't sound right. But everything else on the page is me, so this has to be me. So that's kind of. What you're talking about is the skills.
Speaker 2:That's one of the big use cases, both for hiring and for internal mobility or upskilling. So I did a case study last year with Manulife and they went and Manulife is a big insurance company, global, and this was in their engineering group. So IT and development the project took them from 1,400 roles to 40, so the biggest pull in the tent to your point was change management, whereas, hey, I know your title is software engineer but you're an analyst and let me show you where we're going with the organization. Or we know you've got your department structured with these titles, but we're moving in this direction and some people it wasn't a bad thing, not all change is bad. It was like, oh, you're actually an engineer, good for you.
Speaker 2:That makes sense, but it was a big project. Consultants travel around the world. That was a change management process Once they got that to 40, then maintenance of that became ongoing.
Speaker 1:The flywheel fed itself.
Speaker 2:But now with technology. So this is, I'm going to say, the promise of large language models. But this is where a lot of people are heading. So I think what would be cool in that example you gave is if, dynamically, those skills were to look at you. I didn't the titles that you used. But if it was like yeah, back in engineer versus software developer versus software.
Speaker 1:So here's your title and here's this job, and you could reflect that.
Speaker 2:Here's how your job now compares to this job regardless of the job title is not mapping that promises.
Speaker 1:That is what I would like to see happen organically, in the background. I'm manually mapping it because there are skills that are required. For example, if an organization is not really language dependent, like they're developing in multiple languages, the job posting will have several. You know Go Lang, java, python, et cetera. What that tends to sometimes translate into is that the candidate must have all of the above, which is really important. What it actually supposed to mean is we really don't care if you're good at Go Lang, good at Python and good at Java, as long as you're good at one of them and we would like to know which one is your preference but we'll take them. But instead what people see is man, I love Go Lang, but I don't know enough about it and I haven't used it at work, so I'm not going to apply, and that's creating that. The other question that I have for you folks that are listening George and I go back I don't know what to 10, 15 years at least. Oh, at least, at least it's longer than that.
Speaker 2:Yeah, so I used to work for, we'll say 10, 15. Yeah, something like that. It's more than a decade. I used to work for this company that was a pioneer in the distribution of jobs and I literally went after George.
Speaker 1:I recruited him. You were a recommendation. Did you know? You were a recommendation from someone, an angel investor that I knew.
Speaker 2:I don't know if I ever told you that I don't think you did yeah.
Speaker 1:So I called this friend, my friend of him, like dude, I need somebody that's like no nonsense, that's going to come in and just hit the ground running and can run sales for a mature enterprise product. And they're like, I can only think of one person that gave me your name and I reached out and the rest is history. And so from there, when we both left that organization. But you left and you started your own thing and you became essentially like I want to say, like the Uber analyst, Like an analyst is not a good title for you. You're the mega analyst of work technology.
Speaker 2:All right, thank you, sure Thanks.
Speaker 1:Because you're not just like out there writing an article or two. I mean, you're evaluating products, You're advising products, You're you know, and people depend on your opinion. So two things related to that. Number one literally put everything I just said away for a moment and pretend that you don't know anything about it on the industry and you walk around. What is the most awesome booth? No judgment about what they do. What are? Just the booth itself? Oh wow, Because these booths are darn cool and last year they weren't this pretty.
Speaker 2:Yeah, that's a hard question for me because I've been running that pitch fast, so I just told you, for like this is you haven't walked around.
Speaker 1:I walked from there to unfair unfair question but but they're like massive, like we got. We got baseball field size boots here.
Speaker 2:OK, I am, but here there's one that looks pretty cool. I am not endorsing this product.
Speaker 1:Not at all, it's just a boot. But I mean like I am literally not endorsing this product.
Speaker 2:It's that's going to get me in trouble. It's Ripley.
Speaker 1:Oh yeah.
Speaker 2:And so they've got that the sphere I just walked by and I'm going to stop. It's like a sphere, and then they have one of those. You see it at all the trade shows now, but it's the sphere around it. That's kind of cool and it ties to the I think thematically to the sphere in Vegas. But one of those camera things that spins around you.
Speaker 1:We're in there, ok.
Speaker 2:But it just looks. You know it's, it's, they have nice colors and it's and that very looks very appealing. But that's good yeah.
Speaker 1:So now put all your knowledge back on the table.
Speaker 2:OK, all right.
Speaker 1:And think about what you've seen or heard pitchfaster, not that in the last week or so here. Conversation wise, what is, what is a truly innovative? It's a tough question because I haven't seen a whole lot of innovation. I've seen a lot of expansion into other areas. I've seen a lot of rebranding. Honestly, I haven't seen a lot of new companies, except for startup corridor Yep, and those are all you know new, but unfortunately it's a small booth that you almost have to go to their website to figure out what they do.
Speaker 2:Right, right.
Speaker 1:So all of what you've taken in conversations and everything is there. Is there something that is innovative? Dare I say, game changing?
Speaker 2:Yeah, in work tech it's, it's, it's been. I'm very, I'm so jaded, charlie.
Speaker 1:I've been, I've been around for so long, and that's what we're talking about is you're the Uber animal, so it's you know all this stuff.
Speaker 2:So it's, it's a tough question. It's also not just about me being jaded, but also I'm going to give you an answer, but I it's also about you know, to your point just to explain for it to stand out for you means yeah.
Speaker 2:Well, but just like to to. So those pitches right that we watched, like this year there were like the finalists, like really tight pitches and like immediately you know what they do, not that? Not, I'm not saying that's where the innovation was, but most of these booths I have no idea what they do when I stand in front of it or even when they talk to me, for four or five minutes.
Speaker 1:I've noticed that, yeah.
Speaker 2:And so so it's, you need to peel back the the layers a little bit. There was one of the startups that and this is really foundational, but I just went over after that they were one of the finalists Aragorn AI and what they, what they've built, is remember back in the day we would have these like hub and spoke like connector models to do integrations across platforms. So they, what they've built, is they've leveraged AI. So they had to build, you know, integration I was talking about this yesterday.
Speaker 2:Yeah that they've leveraged AI. Yeah, to do a lift and shift the ETL, yeah, yeah, yeah, that's a really good I was talking about that being a really good application for AI, and that's what they're doing.
Speaker 1:That's yeah, they're doing a lot of higher them to be that I'm going to use a really old word, middleware. Yeah, yeah absolutely the translator between Product A and.
Speaker 2:Product B yeah, they're like the next wave, like beyond. You know we're getting past like the. I'll call it manual, but you know the hub and spoke sort of connector model, or like Zapier, or yeah, yeah and then what that does for the ecosystem. Here is you know you could be on Workday or you could be on Seridian and you could have Greenhouse or whatever you have and the because it's more fluid right, the automation, the opportunities to do interesting things with your data and your workflow is way better than a hard-coded, you know.
Speaker 1:Or you have to write a custom API or hire yeah, you got to go back, yeah, we want to do this now. Software engineering, do the integration specifically for your version of whatever. Because everybody you know, ultimately, because not very many maybe in your experience but in mine not very many organizations will like kind of use the out-of-the-box. Yeah, they'll, you know.
Speaker 2:Yeah, I'm going to. I'll give you another startup answer To Argonne.
Speaker 2:Yeah yeah, eric Gorn, eric Gorn, Eric Gorn, ai. Yep, then I can't remember the name of this company because it was one of the 33 pitches, but this is a creepy innovation. They did a facial recognition. Like you're sitting at your desk and this thing is on all the time and doing facial recognition and measuring employee sentiment. You know they're like claiming to measure, like you know, mental wellness, and I was the host so I didn't get to ask all the questions that I had but that's I think that's a really scary place to go.
Speaker 1:It's innovative, I'm assuming that they're not recording but analyzing, right, because that would be kind of the so you know, first of all, recording massive amounts of video and story is like kind of useless but also the privacy infringement in that, you know, even if you're at work, the security camera in your office might record on a loop, but it's not intended to record permanently.
Speaker 1:So let's assume that they're essentially analyzing, you know, overall wellness based on your facial expressions or whatever right? So they're analyzing the data. I can see the applications on that, but I can also see how that would be. You know, you know my background.
Speaker 1:My undergraduate is in intercultural and non-verbal communications and to me one of the biggest mistakes that we make, generally speaking as humans, is that we misinterpret what other people are thinking or feeling based on what our particular proclivities might be Right. So, for example, I'll just give you a really easy example you and I being from a Western culture, to us a smile that is a nice big smile, is sort of toothy. Right, you open up wine, you show your pearly whites and that's a very friendly, warm smile, where there are cultures where if you show your teeth is an act of open aggression. So my concern with this intelligence is that if it doesn't detect toothy smiles, it's gonna say, oh, this person's unhappy, when in fact it might be that they're quite happy. They're just culturally predisposed to not, you know, have a toothy smile, or it's just one thing. And then you've got neurodivergent people and you've got people who have facial tics. I mean, I'm thinking about all the possible misinterpretations that could happen there?
Speaker 2:Yeah, I think there. Yeah, I wasn't surprised to see it, given you know.
Speaker 1:AI, all the AI hype and everything.
Speaker 2:Here's another one. You might like. This one collab work that sounds familiar. They do non-traditional posting of jobs and recruitment, so what they call the hidden talent. So they're not going to job boards, they're not going to social media, they're getting jobs out into Slack channels and they're getting into other destinations where the talent that you're looking for is not necessarily there.
Speaker 1:It's not about jobs, Hidden talent, not intentionally hidden, but yeah and that's the phrase they use.
Speaker 2:You know, they've got AI, you know, and everybody's got AI in the mix. I'm not sure how they're using it, but it was pretty, I think, with all the pressure on finding, finding talent through new channels.
Speaker 1:Just tab sources.
Speaker 2:I think you know I think they'll definitely get some traction.
Speaker 1:For sure I can think of applications for that in very niche areas where you don't really have people looking on the traditional job boards because they know that there's not a lot of jobs for them.
Speaker 2:Yeah, I mean I go back when I was recruiting. I don't know if you remember the Usenet news group days, yup, usenet, I mean I got a lot of good candidates out of Usenet. Yeah, they'd sit there on the Mac and, just you know, put the job every day, set aside an hour to get the jobs. That was the old boards.
Speaker 1:Yeah, oldest one of those would probably be like the well, from back in the, you know you dial up days, but you know. So you've mentioned AI a couple times and I know it's one of our bingo words, yeah. Yeah, I would love for you to explain what, in your perception, is really called AI, because I know, and you probably know that I know that it's not AI, right, it's not really true AI. So they call it generative AI because they want to qualify it and you know, basically just large language models and it's data science and it's machine learning. But what, in your estimation, as an analyst, looking at this industry deeply, what you know? They're calling it AI. They used to call it semantic search. They used to call it, you know, whatever, but what is it really?
Speaker 2:Yeah, and I think you bring up a really good point. If you know it could be machine learning, it could be. There are so many flavors of what's what they call it yeah, the AI is like the catch all term. Yeah, I think at an event like this, I think that's probably a good way to look at it.
Speaker 1:It's a catch all term.
Speaker 2:It's more of a catch all term. I think what generative AI did was open up everybody's minds to the possibilities, and a lot of what we have out there is aspirational. It's, you know, super aspirational right now. I don't think, you know, I don't think there's anything on this floor that's going to pass the Turing test yeah. So you know, there's Right right.
Speaker 1:So you know the traditional definition of a true artificial intelligence that's able to extract subtext from context and can make sense of a variety of different things. You know that's not what we're looking at here. That's what I was kind of wondering is if you have a sense of what, in general, they're referring to when they say AI. Is it, generally speaking, the you know large language models, or is it something else? Because the video processing one they're doing, that's not large language models. Right, and the you know the other ones that I've seen on here that are more around like chat bots. That's also not really a large language model. There's a gentleman that was at the podcast earlier talking about recruiting sourcing tool that they're using. He's a data scientist and what they've created is not a large language model, the type that you would see in chat GPT, for example, which is unhomogenous data. His is specifically homogenous. So you used to be a board member of the HRXML consortium.
Speaker 2:Right right.
Speaker 1:Literally, what is the HRXML equivalent these days? You know that doesn't exist anymore. No, no. But what is the? Are these language models? Essentially a new way of sort of categorizing data into some sort of Homogenous pool so you can later use it Like. These are conversations, these are resumes, these are, you know, is that what we're talking about? Because machines can do that really well.
Speaker 2:I think some of them are talking about that. So like an eight-fold they before they ever came out with anything generative they put a lot of effort into large language models. Their founders are, you know, scientists from Google.
Speaker 1:Data science. Yeah, so that's an ontology, a taxonomy. Yeah, exactly that's what semantic search used to be.
Speaker 2:Right, and so you look there and I would say there's an example. They're doing that. Now, your average recruiting tool that is talking about AI is probably got a field or an area in the app where it'll write an email for you. It's using generative AI and it's using not their own large language model.
Speaker 1:No, they're borrowing from somebody else's which is scary because it becomes everybody is using the same language.
Speaker 2:It's very bland.
Speaker 1:Right, Very middle of the road, which is not very convincing yeah now others are.
Speaker 2:You know, back to the skills conversation or if you're thinking about something that's a little more sensitive, like when you're talking about job descriptions, those are going external and those are exposed anyhow so you don't have to be as careful with those, and it's good to capture more of a global sort of learn from the world at large, but if you're looking at anything close to or involving PII or sensitive data, you need to have enough data to have your own language model, and it's got to be private, otherwise you're exposing a lot of risk.
Speaker 2:So there are a lot of and don't get me wrong a tool that makes your email sound better, that engages candidates better, that's effective.
Speaker 1:And that's really helpful. Most of us are not great writers.
Speaker 2:Yeah, so that's great, but that's becoming table stakes, like really fast.
Speaker 1:That's my point.
Speaker 2:That is table stakes. Now, like every tool that I use I'll say almost every tool that I use on my desk has something like that.
Speaker 1:Yeah, there's something you know it's an assistive thing. Yeah, it's clippy, it's clippy.
Speaker 2:Yeah, it's just yeah, remember clippy. Yeah, I do remember clippy, it's clippy.
Speaker 1:Yeah, okay, so that makes me feel a little bit better about kind of the state of the industry, because then we have a lot of different places to go from here rather than just everybody calling it, because it used to be all everybody called it semantic search and we wrote that white paper and then people stopped talking about it and now it's this other thing and you know, probably gonna be the buzz for a while. People stopped talking about it. It kind of gives me the you know, social media, Way back when we started talking about social media and we were talking about, ooh, social media, now it's just media man.
Speaker 1:I mean it's not social, it's just, it is part of the mix. So AI is going to be somewhere along the line part of the mix.
Speaker 2:Yeah, I think that's an excellent analogy and I actually use that example a lot. And so we used to talk about social recruiting and it's just recruiting now and it took about 10 years to really we had to become mainstream and we had success and we had all along the way, but it took seven to 10 years. I'll say before you really, mobile recruiting, right, yeah, right, mobile, yeah.
Speaker 1:So this will be AI in five years. It'll just be recruiting, yeah, I think yeah.
Speaker 2:So this cycle, I think, is more like five years on the money and another thing that should make you feel good. So I just in the recruiting space, specifically recruitment marketing space, I've been on a big journey, talking to everybody, looking at because there are a lot of threats around with what's coming here, and I ventured outside. I went and said, well, what are they doing in B2C marketing and in B2B marketing? So I tapped into thought leader stuff there. They sound just like us. They have the conversation we just had.
Speaker 2:We could have been talking about marketing platforms and CRMs in B2C in B2B and so with that, when I say it should make you feel good, we're all in it. Yeah, well, we're on par. So we always used to say we're five to 10 years behind B2B and B2C, maybe not this time.
Speaker 1:We're not, we're not, yeah, maybe not this time.
Speaker 2:In some areas we may be when you're talking about the more true AI that you're poking around, but I'm talking about the application of this stuff on user desktop. We're neck and neck with everybody. It's exciting, it's really exciting.
Speaker 1:It is. Yeah. Well, you know, what really makes me feel really good is to have you here and see you. It's been a few, quite a bit, since we last got a chance to talk and, yeah, I'm really happy you came by.
Speaker 2:Thanks, it was great to see you last night for a few minutes and then, yeah, to chat. This was a bonus.
Speaker 1:So here we are wrapping up at the Oleo booth, the HR Tech Expo with WorkTech. George Larac, Thank you very much.
Speaker 2:Thank you, thanks for having me and thanks everybody for listening Take care bye-bye.